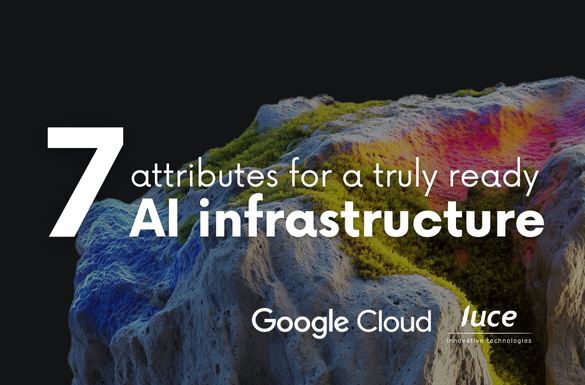
7 key features for a truly AI-ready infrastructure
The speed at which generative artificial intelligence is advancing is staggering. And what is even more impressive is that it has only just begun. Organisations that want to remain competitive in this new era must make strategic decisions today, especially when it comes to their technology infrastructure.
Google Cloud asked more than 500 technology leaders around the world how they are addressing this challenge. The result is clear: success in AI is not achieved by simply adding an extra workload, but by building a solid and flexible foundation capable of sustaining the specific demands of this ever-evolving technology.
Today you decide what your future in AI will look like
The conclusion is clear: anyone who wants to be prepared for an AI-driven future needs a robust, scalable and well-integrated platform. We are not just talking about tools, we are talking about a complete infrastructure that enables AI models to be trained, deployed and managed efficiently and securely.
A stable and powerful AI platform is a priority for 45% of respondents. Not surprisingly, the volume of requests these systems must handle – including communications between AI agents and real-time inference processes – is growing.
As a result, performance and scalability have become critical factors everywhere in the world.
What should an AI platform have?
A well-designed infrastructure will allow you to adapt quickly to new needs and lead with confidence in an increasingly demanding environment. What should you look for? Here are 7 key attributes:
1. Secure
Security and compliance are not optional. With data spread across multiple systems and formats, achieving a unified security policy is difficult. This is why it is key to have a single platform that unifies all data – even in open formats – and allows data to be kept secure, private and compliant with current legislation.
2. Scalable
A scalable architecture allows you to combine transactional and analytical systems without sacrificing performance. This facilitates data access between different environments, even under demanding workloads.
3. Optimised for storage
Advances in AI have brought new types of storage to the forefront, such as those that leverage GPUs for edge environments. But it’s also important that your infrastructure can adapt to each use case, combining CPUs, custom silicon and different storage systems (files, objects, blocks) to maximise efficiency and performance.
4. Dynamics
The platform must evolve as generative AI evolves. For example, variable-length contexts require more intelligent load balancing, and multi-step reasoning may require multiple distributed computing resources. Flexibility is key.
5. Ready for the edge
More and more AI applications are running outside traditional data centres. Autonomous vehicles, IoT or mobile devices need to process information in the moment. This requires efficient and well-managed resources at the edge, with cloud solutions that simplify real-time deployment.
6. Hybrid
73% of organisations opt for a hybrid strategy that combines on-premise resources with cloud services. This allows you to choose the ideal environment for each workload, but also requires you to think carefully about how you manage performance, security and costs in each case.
7. Managed
Many businesses – and governments – do not have the time, resources or talent to develop these technologies on their own. As a result, it is increasingly common to rely on managed service providers that can deploy, scale and secure the entire infrastructure with agility.
What about costs? It all starts with infrastructure
While generative AI promises productivity improvements and savings, it can also drive up costs if not well planned – from compute usage to data management to model maintenance.
83% of technology leaders surveyed say cost efficiency is a critical factor when evaluating AI infrastructure solutions. To achieve this, they recommend focusing on three key areas:
- Precise adjustment of resources: Platforms that allow you to automatically scale and adjust computation according to the real needs of the moment. This way you avoid over-provisioning and only pay for what you use.
- Hardware and software optimised for AI: Use specialised accelerators (such as TPUs or GPUs) and frameworks such as JAX, TensorFlow or PyTorch, which take full advantage of the hardware and accelerate training and inference.
Intelligent resource management: From dynamic load scheduling to the use of pre-emptable instances or advanced caching systems. It all adds up to reduced downtime and better utilisation of infrastructure.
The cloud partner becomes a strategic ally
Having a trusted cloud provider is a strategic decision for any organisation that wants to successfully meet the challenges of AI – from development to deployment to efficient resource and cost management.
To be truly ready, you need an infrastructure that is built from the ground up for AI, but also supports traditional applications and distributed loads. In short, a solution that is secure, flexible, efficient and designed to scale.
Is your infrastructure ready for the AI era?
Read the full report on the state of cloud infrastructure in the era of generative AI to find out how the market is positioning itself and where the opportunities for improvement lie.
Luce IT, your trusted technology innovation company
The Luce story is one of challenge and non-conformity, always solving value challenges using technology and data to accelerate digital transformation in society through our clients.
We have a unique way of doing consulting and projects within a collegial environment creating “Flow” between learning, innovation and proactive project execution.
At Luce we will be the best by offering multidisciplinary technological knowledge, through our chapters , generating value in each iteration with our clients, delivering quality and offering capacity and scalability so they can grow with us.